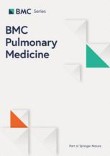
Mochizuki, H., Hirai, K., Furuya, H. et al. BMC Pulm Med 24, 394 (2024). https://doi.org/10.1186/s12890-024-03210-7
Abstract
Background
Lung sound analysis parameters have been reported to be useful biomarkers for evaluating airway condition. We developed an automatic lung sound analysis software program for infants and children based on lung sound spectral curves of frequency and power by leveraging machine learning (ML) technology.
Methods
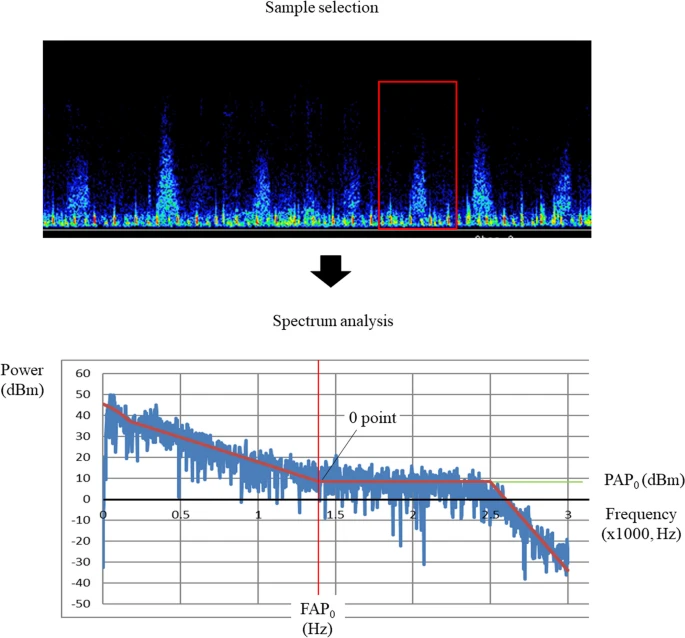
To put this software program into clinical practice, in Study 1, the reliability and reproducibility of the software program using data from younger children were examined. In Study 2, the relationship between lung sound parameters and respiratory flow (L/s) was evaluated using data from older children. In Study 3, we conducted a survey using the ATS-DLD questionnaire to evaluate the clinical usefulness. The survey focused on the history of wheezing and allergies, among healthy 3-year-old infants, and then measured lung sounds. The clinical usefulness was evaluated by comparing the questionnaire results with the results of the new lung sound parameters.
Results
In Studies 1 and 2, the parameters of the new software program demonstrated excellent reproducibility and reliability, and were not affected by airflow (L/s). In Study 3, infants with a history of wheezing showed lower FAP0 and RPF75p (p < 0.001 and p = 0.025, respectively) and higher PAP0 (p = 0.001) than healthy infants. Furthermore, infants with asthma/asthma-like bronchitis showed lower FAP0 (p = 0.002) and higher PAP0 (p = 0.001) than healthy infants.
Conclusions
Lung sound parameters obtained using the ML algorithm were able to accurately assess the respiratory condition of infants. These parameters are useful for the early detection and intervention of childhood asthma.