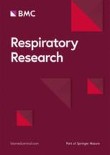
Tian, J., Zhang, Q., Peng, M. et al. Respir Res 26, 173 (2025). https://doi.org/10.1186/s12931-025-03242-5
Abstract
Background
Globally, chronic respiratory diseases have become the third leading cause of death, including chronic obstructive pulmonary disease (COPD) and asthma, and have been threatening human life for a long time. To alleviate the disease burden, it is crucial to develop rapid and convenient screening methods for COPD, preserved ratio impaired spirometry (PRISm), and asthma. Volatile organic compounds (VOCs) in breath can reflect the pathophysiological processes of disease, thereby having the potential to serve as a promising approach for diagnosing respiratory diseases. Can we identify VOC markers in breath with the potential to serve as classification indicators, and further establish learning models for the early detection of COPD, asthma, or PRISm patients?
Methods
This is a cross-sectional study in which exhaled breath samples were collected from 184 patients with COPD, 66 patients with asthma, 72 PRISm individuals, and 45 healthy individuals. From August 2023 to June 2024, the breath samples were analyzed using portable micro gas chromatography (CXBA-Alpha, ChromX Health Co., Ltd.). Potential VOC markers for classification were identified by univariate and multivariate analyses. Subsequently, classification models were established by machine learning algorithms, based on these VOC markers along with baseline characteristics. The sensitivity, specificity, and accuracy of these models were calculated to assess their overall discriminatory performance.
Results
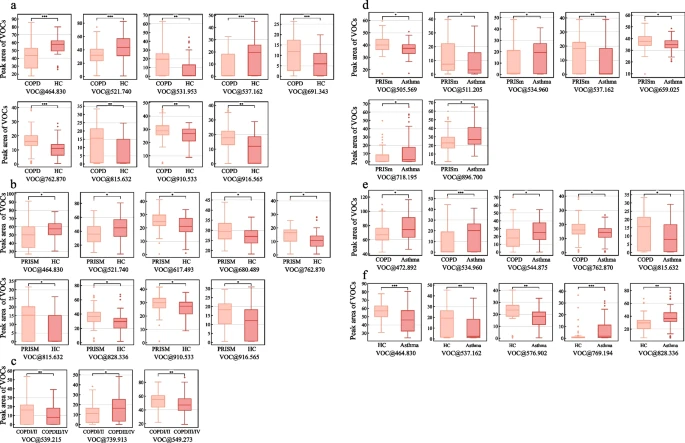
A total of 367 patients were enrolled in our study. We identified nine VOCs distinguishing COPD patients from healthy controls, nine VOCs differentiating the PRISm population from healthy controls, five VOCs separating asthma patients from healthy controls, five VOCs distinguishing COPD patients from asthma patients, and seven VOCs differentiating the PRISm population from asthma patients based on breathomics feature selection. We utilized five algorithms to establish diagnostic models and selected the optimal one among them. The random forest model best distinguished COPD from healthy controls with an area under the receiver operating characteristic curve (AUC) of 0.92 ± 0.01. The support vector classifier (SVC) model was most effective in separating PRISm from healthy controls, achieving an AUC of 0.78 ± 0.01. Logistic regression performed well in discriminating asthma from PRISm (AUC, 0.74 ± 0.02) and COPD (AUC, 0.92 ± 0.01), in contrast, the random forest model differentiated asthma from healthy controls with an AUC of 0.81 ± 0.02.
Conclusion
VOC panel-based classification models have the potential to be a novel strategy for the discrimination of chronic respiratory diseases. Using the portal micro gas chromatography enables swift detection of chronic respiratory disease and, most importantly, facilitates the rapid identification of PRISm individuals within the population.
Highlights
1. We have identified a group of volatile organic compounds present in exhaled breath that can be used to distinguish between patients with COPD, asthma, PRISm individuals, and health individuals.
2. This study pioneers a breathomics-based rapid identification method for PRISm individuals, offering a novel strategy for early detection and management of pre-chronic obstructive pulmonary disease.